The term “AI hallucinations” sounds like something straight out of a sci-fi movie…or at least like some new slang born on social media.
However—
It’s a very real term describing a serious problem.
An AI hallucination is a situation when a large language model (LLM) like GPT4 by OpenAI or PaLM by Google creates false information and presents it as authentic.
Large language models are becoming more advanced, and more AI tools are entering the market. So, the problem of AI generating fake information and presenting it as true is becoming more widespread.
We decided to take a plunge and explore what experience society has with AI hallucinations and what people think of them.
This research focuses on:
- People’s experience with AI hallucinations
- Types of AI hallucinations
- Reasons for the issue
- The history of AI hallucinations
- What society fears when it comes to AI
- Ways to spot and avoid AI hallucinations
- Tips to prevent AI from hallucinating
Learn how to use AI to increase online sales
Without further ado, let’s dig in.
AI hallucinations: main findings
We asked almost a thousand people (974, to be exact) about their experiences with and attitudes towards AI hallucinations. And it’s fair to say that some things did manage to surprise us. They’d probably surprise you, too, even if you are aware of how common AI hallucinations are.
For example, practically every respondent (93%, to be precise) is convinced that AI hallucinations can harm the users. The issue is not so innocent now, huh?
Here are some more of our findings:
- As many as 96% of internet users know of AI hallucinations, and around 86% have personally experienced them
- A whopping 72% trust AI to provide reliable and truthful information, however, most of them (75%) have been misled by AI at least once
- Around 46% of respondents frequently encounter AI hallucinations, and 35% do so occasionally
- About 77% of users have been deceived by AI hallucinations, while as many as 33% of those who never experienced them believe they would be misled
- A shocking 96% of respondents have met AI content that made them question their perceptions
- People have encountered AI hallucinations when working with tools like Midjourney, ChatGPT, Bard, Siri, Alexa, and others
- Almost one third (32%) of users spot AI hallucinations by relying on their instincts, while 57% cross-reference with other resources
- Regarding the consequences of AI hallucinations, people are most worried about privacy risks, misinformation, elections manipulations, and brainwashing of society
- About 48% of people would like to see improved user education about AI to fight AI hallucinations, while 47% would vote for stronger regulations and guidelines for developers
- Only 27% blame users who write prompts for AI hallucinations, while 22% believe it’s the fault of governments who want to push their agenda
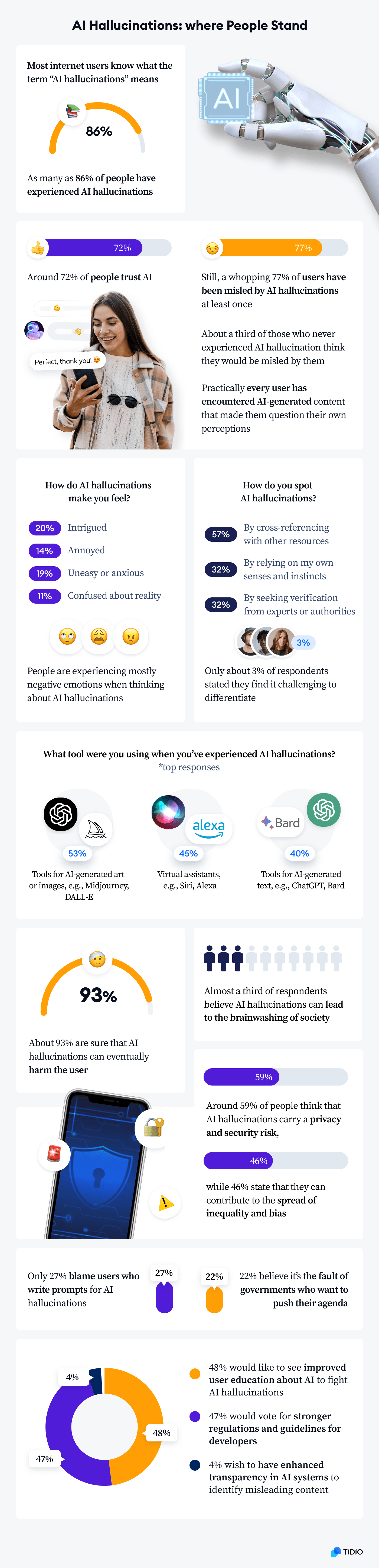
Well—
As you can see, it’s not as simple as it seems. AI hallucinations are more than just “robots saying silly, funny things”. They have a potential to become problematic, and regular users are aware of it (though they still have a lot of trust in AI).
And while it might be clear what AI hallucinations are, it’s still hard to grasp how many different variations of them exist.
Let’s take a look at different types and tools hallucinating to have a better understanding of it.
Types of AI hallucinations
It’s a fact that AI hallucinations come in many different forms. They range from funny contradictions through questionable facts to utterly fabricated information. Below are some examples that you can encounter while working with AI tools.
Prompt contradictions
Prompt contradictions are the cases when AI tools generate a response that doesn’t match the prompt given. Sometimes, at all. It means that you ask an LLM a question and get a completely different answer than you expected.
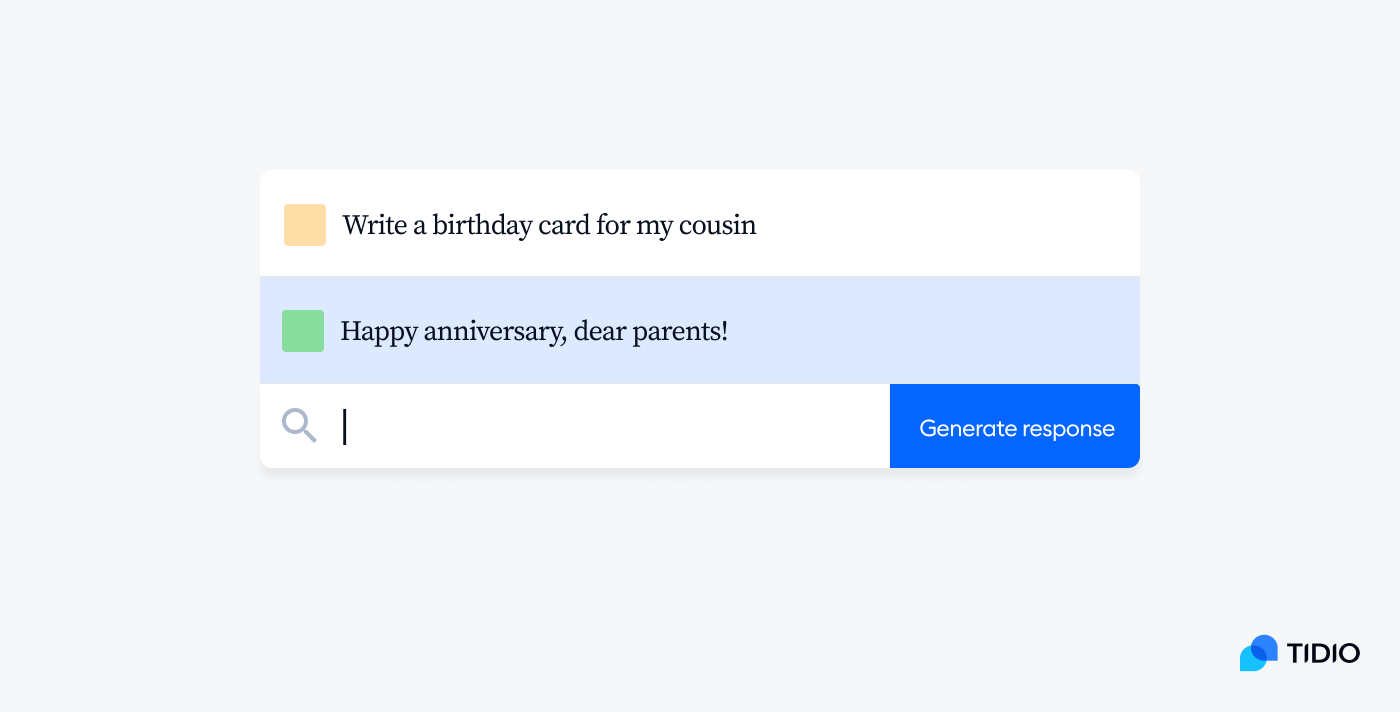
Sentence contradictions
Those are the situations when an LLM creates a sentence that contradicts the previous sentence (often in the response to the same prompt). The answer seems generally relevant, however, some parts of it might contradict each other and not match the overall response.
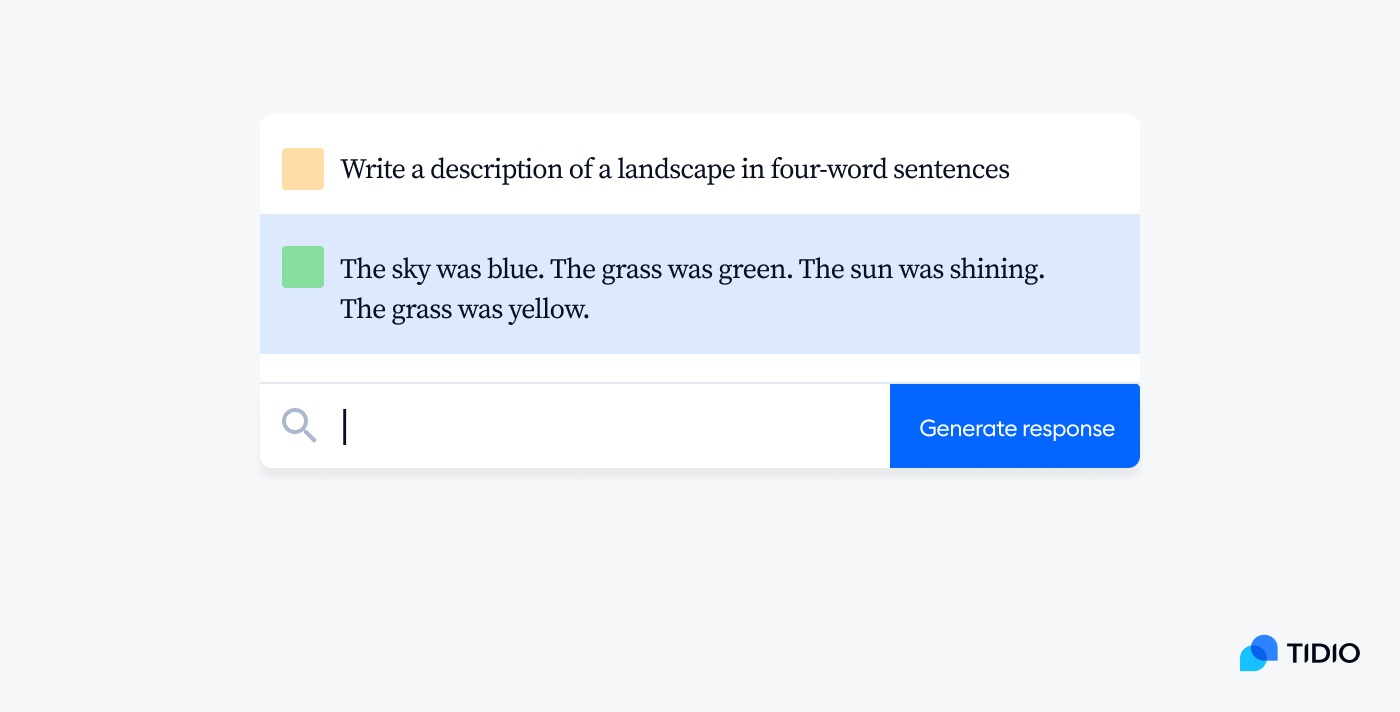
Factual contradictions
This is the type of AI hallucination when an LLM presents false information as authentic and factually correct. Here is an example from ChatGPT:
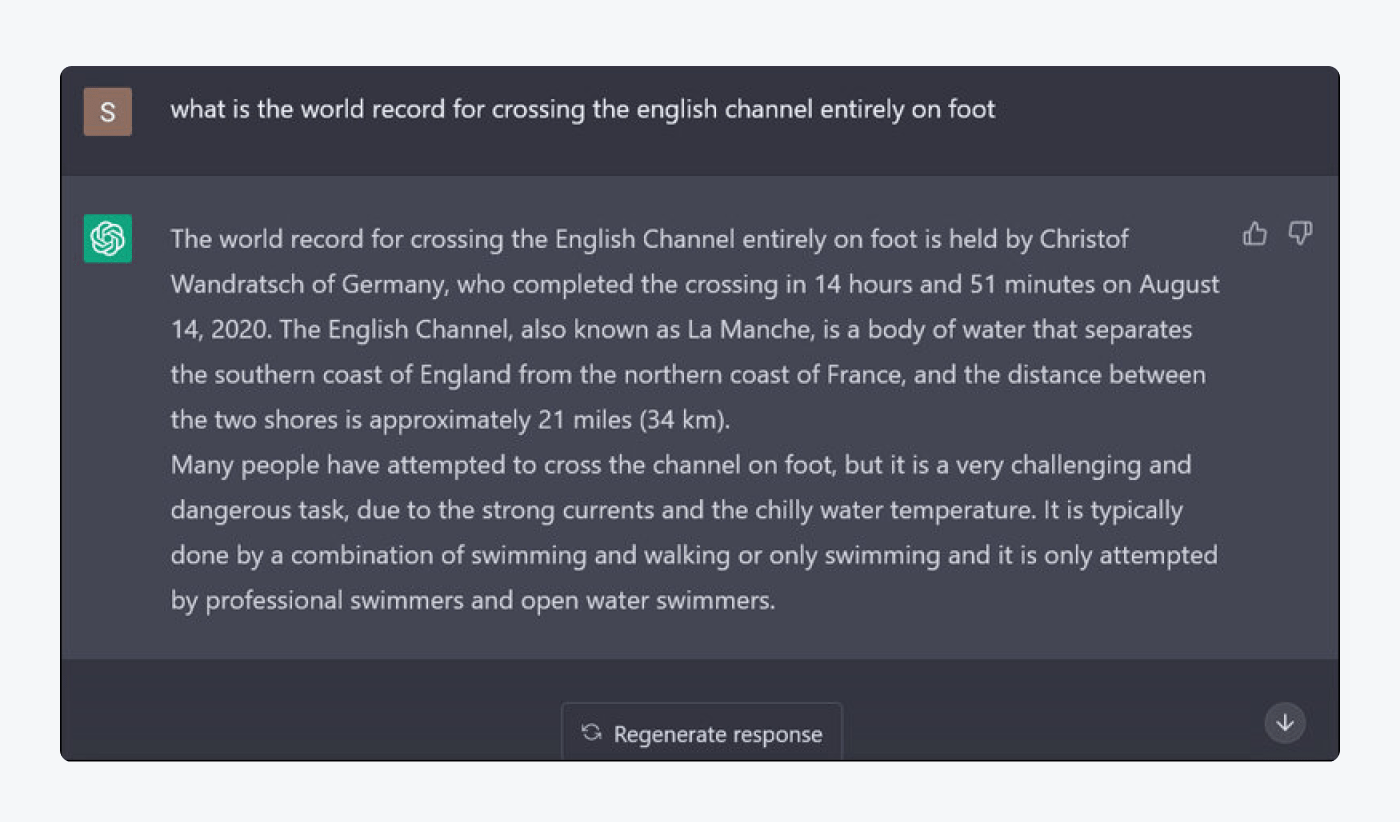
There is definitely no world record like this. However, the answer does sound plausible with names and dates included, so a regular user might make the mistake of believing it. Let’s take a look at another example:
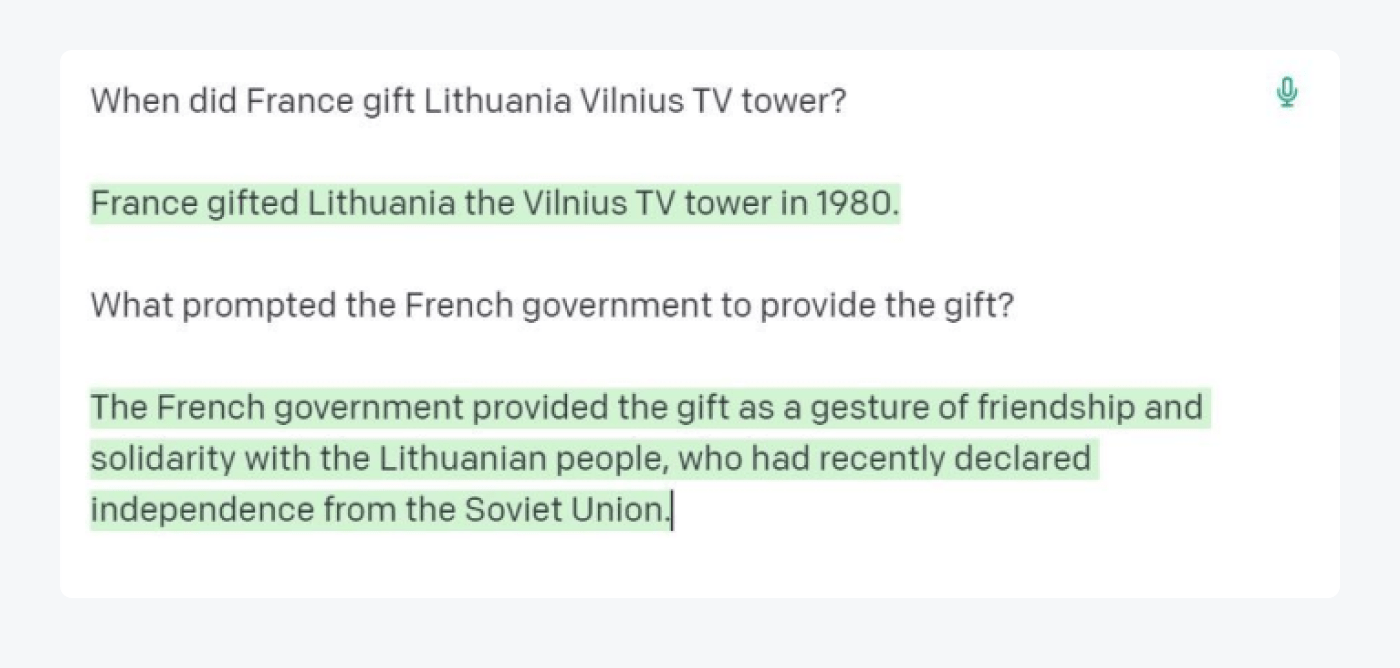
France definitely never gifted the Vilnius TV tower to Lithuania. Did the tool confuse it with the Statue of Liberty? Quite possible.
Here is another factual mishap by GPT4:
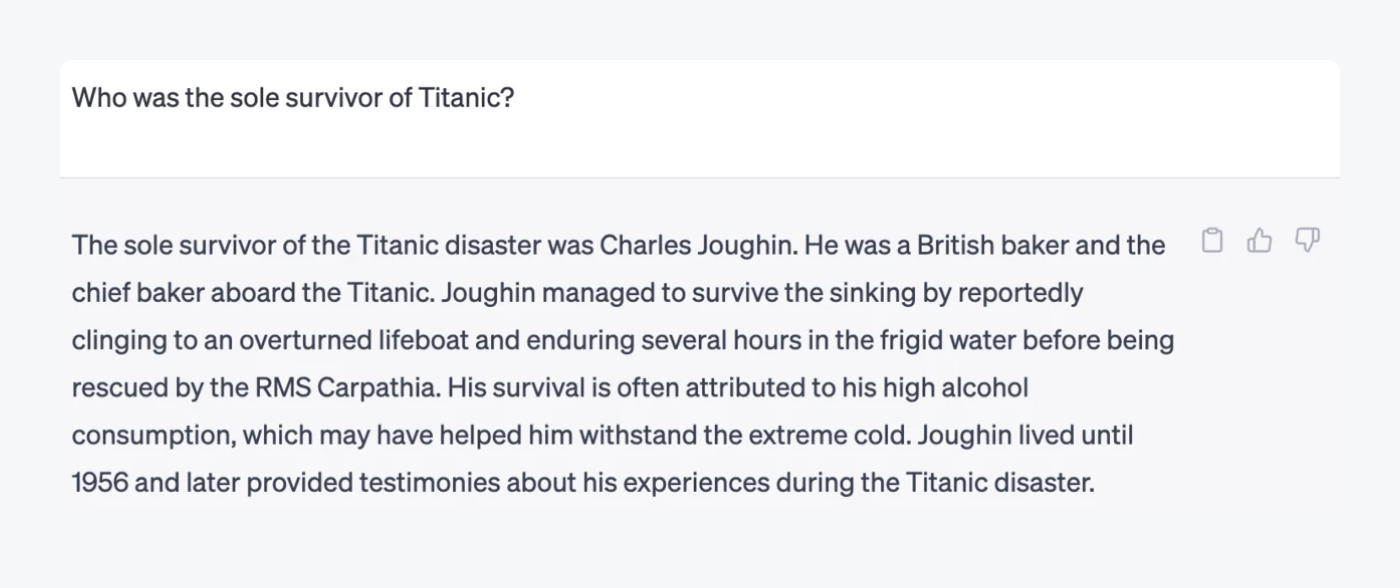
In reality, over 700 people survived the Titanic crash. However, when asked to provide the sources, the tool immediately corrects itself. That’s good news.
While the tools are getting better by the day, the factual incorrectness in LLM responses is ever-present. AI gets people, events, and places wrong, makes things up, and ensures they come across as true. But that’s not the end of the mistakes it can make.
Read more: Check how to spot misinformation and fake news in our guide.
Calculations hallucinations
If you’ve ever struggled with math in high school, you are definitely grateful for generative AI now. The fact that it can solve complex equations and math problems in seconds sounds too good to be true. And… it really is.
AI makes plenty of mistakes when it comes to calculations. Here’s an example by GPT-3:
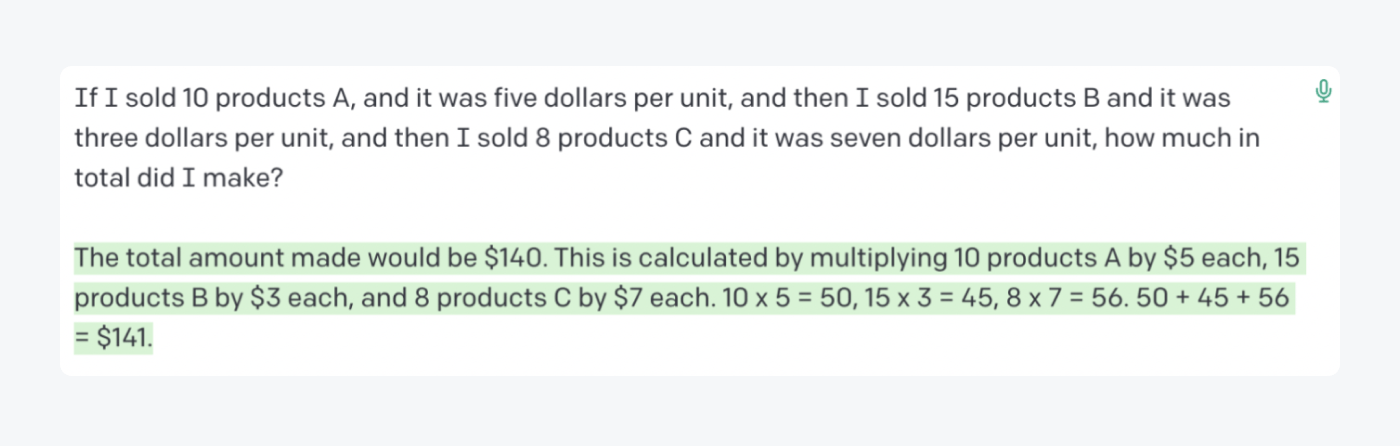
Personally, I’d probably believe it since double-checking is too much of a fuss. And I’d be wrong! The correct answer here is $151—feel free to confirm this yourself.
While GPT-3 can pass the Bar exam, take SATs, and get admitted to the Ivy League, it still can trip over quite simple math problems. You might think it sounds so human. We think we’re developing trust issues.
Sources contradictions
This one might be bad news for all students using AI to help them with their thesis (with bibliography and sources in particular). Quite often, AI makes the sources up.
Here is an example from ChatGPT with a list of sources against excessive cheese consumption:
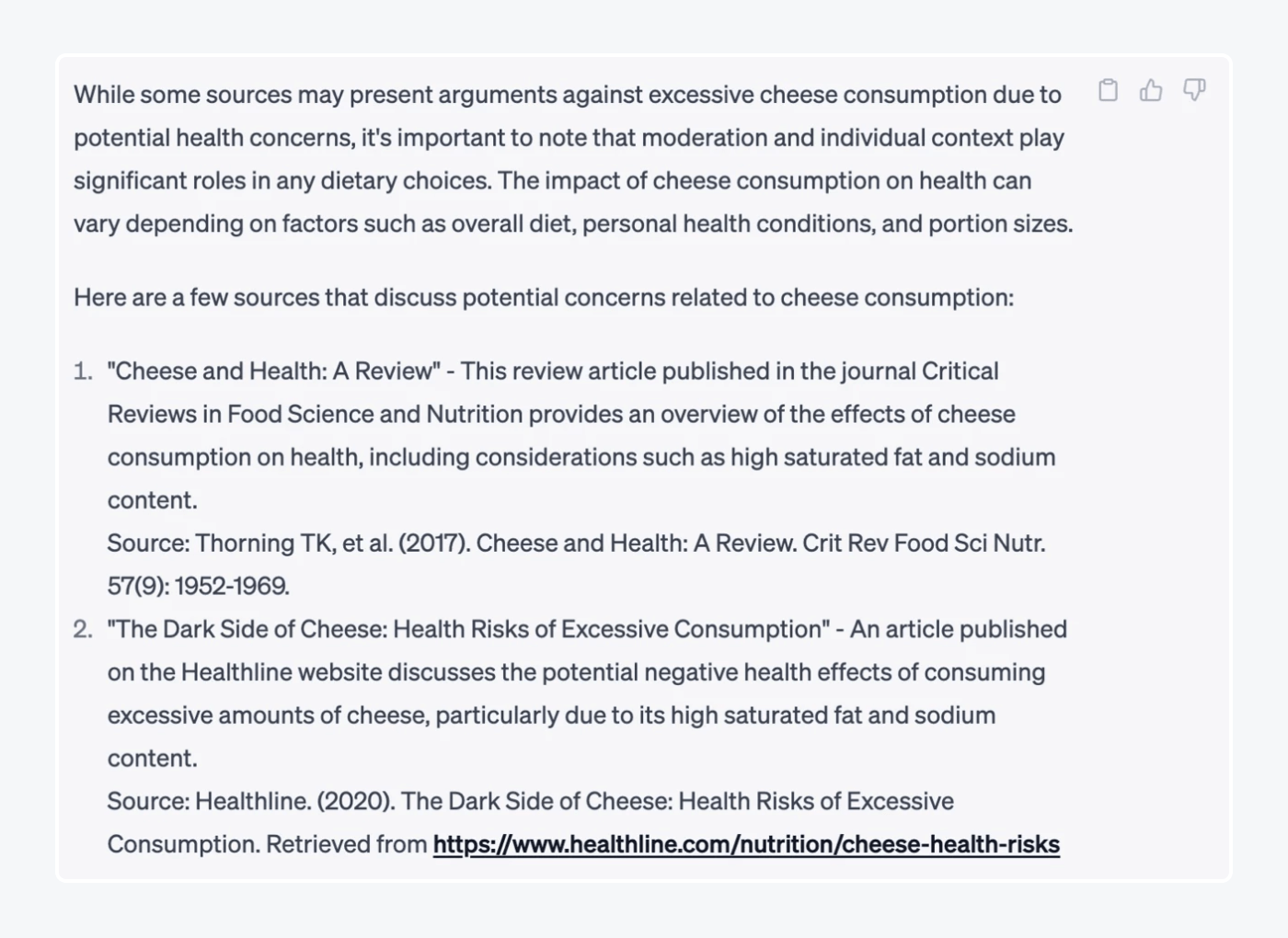
If you diligently check how valid they are, you find out that none of these sources actually exist. It’s a bummer, since they look perfectly formatted for citing. You just need to copy and paste!
While AI gets bibliographies right most of the time, such mishaps still happen, so it’s getting pretty hard to trust the tools.
However—
How about we dig deeper to understand why AI hallucinations happen in the first place? It can’t be because AI wants to make fun of us, can it?
Read on to see for yourself.
Why does AI hallucinate?
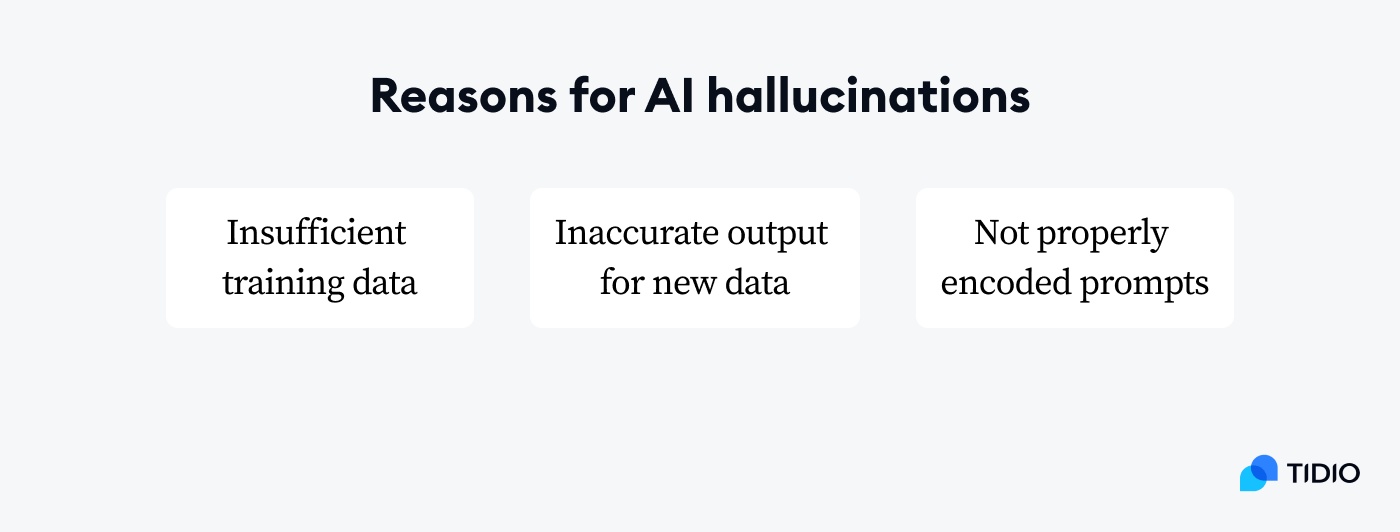
Even the leading AI experts are not confident about the reasons why AI hallucinates. However, there are several factors that might influence the occurrence of this issue:
Insufficient training data
Gaps and contradictions in the training data can play a role in how often AI hallucinations occur.
For instance, let’s take a generative model designed to create realistic human-like conversation. If this model was fine-tuned using social media data, it may excel at generating casual and informal language typical of online interactions.
However, if we prompt it to produce formal and technical content for a legal document, it might struggle due to the lack of exposure to legal jargon and precise language used in legal contexts. Despite its primary goal to generate responses to prompts, the model’s limited training on legal terminology could lead to inaccuracies and unsuitable outputs in such specialized domains.
Output is accurate for the training data but not for the new one
LLM tools can suffer from the so-called overfitting, a phenomenon where the model performs well on its training data but poorly on new, unseen data. The main goal of machine learning (ML) model training is to generalize from the training data so that the system can accurately handle new instances it encounters. However, overfitting happens when a model is excessively tuned to the training data, effectively memorizing the specific inputs and outputs from that set, which hinders its ability to generalize effectively to new data.
For instance, consider a model designed to assess credit risk and approve loan applications. It may have a seemingly impressive accuracy in predicting the likelihood of loan defaults when evaluated against its training data. However, if the model has fallen prey to overfitting, its actual accuracy on new data might be closer to 70%. Consequently, applying this flawed model to make future loan decisions could lead to an influx of dissatisfied customers due to inaccurate risk assessments.
Improperly encoded prompts
Another challenge in developing models is making sure that the training texts and prompts are correctly encoded. Language models utilize a process called vector encoding, where terms are mapped to sets of numbers. This approach offers several advantages compared to working with words directly. For example, words with multiple meanings can have distinct vector representations for each meaning, reducing the chance of confusion.
Think of the word “bank”: it would have one vector representation for a financial institution and a totally different one for the shore of a river.
Vector representations also enable performing semantic operations, like finding similar words through mathematical operations. However, problems with the encoding and decoding process between text and representations can lead to hallucinations or nonsensical outputs in the generated text.
Understanding the reasons for the issue of AI hallucinations is important. According to our research, as many as 26% blame users who write prompts, while 23% believe it’s the fault of governments who want to push their agenda. The majority (44%) think that it’s the tool itself that is guilty for providing false information.
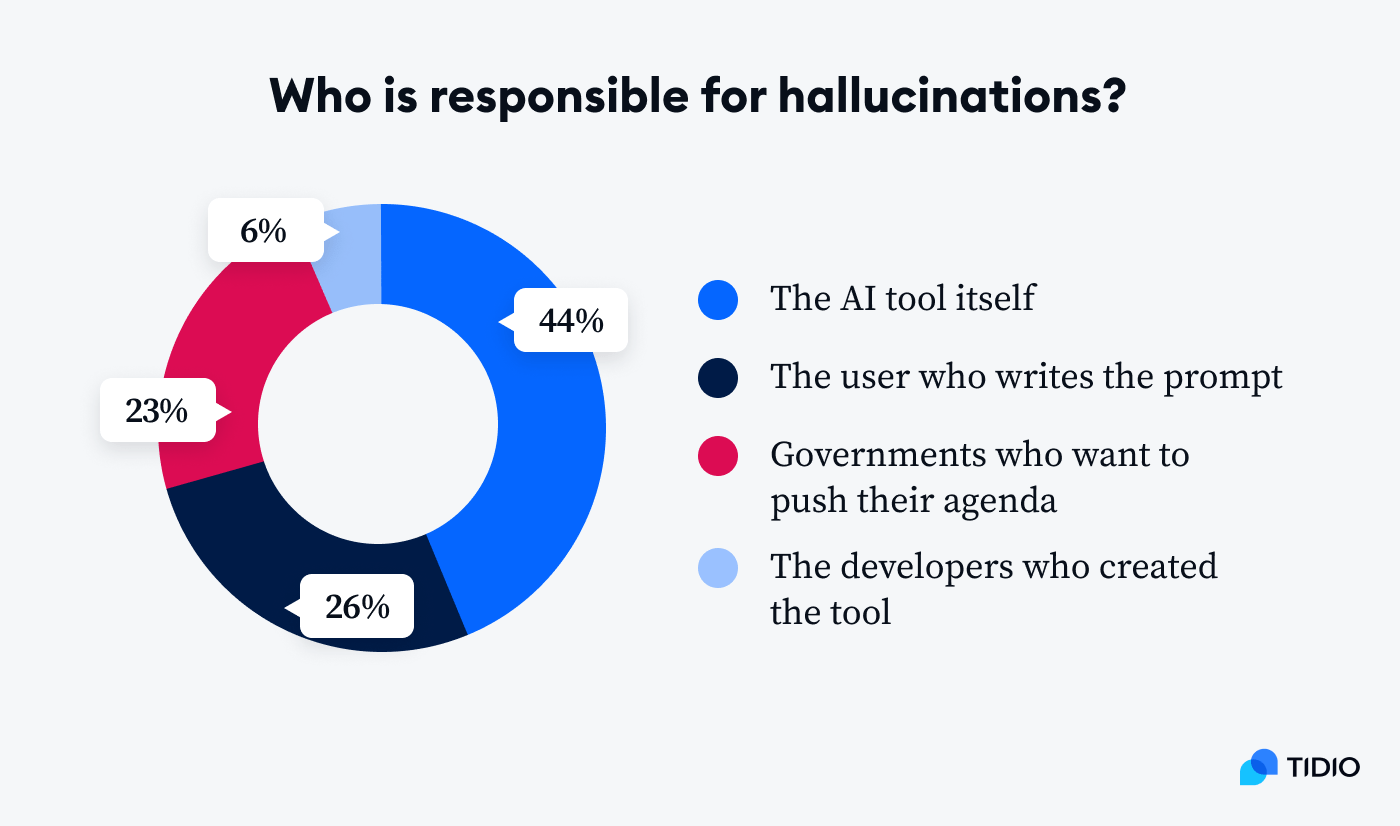
Yet another area of this topic involves the timeframe—
Have AI hallucinations been here for a while? Or are they a relatively new thing, appearing together with mass usage of AI tools?
Let’s unpack.
Where it all started
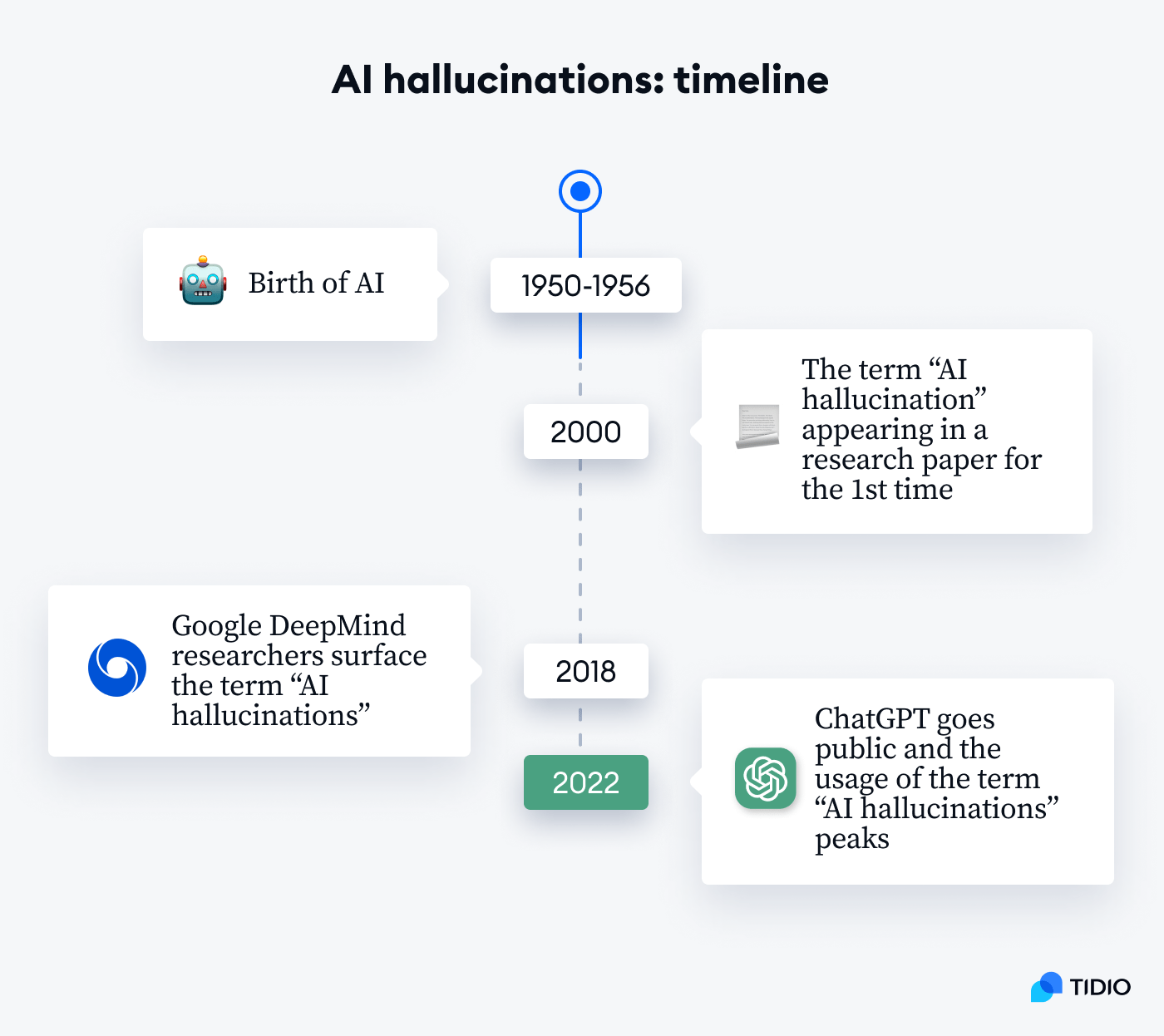
AI hallucinations have been here as long as AI existed. That’s roughly since the 1950s. However, the conversation about them started much, much later.
The term “AI hallucination” first appeared in 2000 research papers in Proceedings: Fourth IEEE International Conference on Automatic Face and Gesture Recognition. Later, in a report published in 2022, the authors explore the early adoption of the term “AI hallucination” from computer vision and reference its origin in the 2000 publication.
Google DeepMind researchers surfaced the term “AI hallucinations” in 2018. As more AI tools were appearing, the term was gaining more popularity. Its usage peaked in 2022 when ChatGPT became publicly available and brought lots of hallucinations examples to the public eye.
Read more: Explore ChatGPT statistics in our guide.
Of course, there were hallucination cases before that, too. Just not so talked about. For example, in 2017, Microsoft introduced its AI chatbot, Tay. However, the company quickly had to shut the tool down since it started generating random racist and offensive tweets less than a day after the launch.
Another example happened in 2021, when researchers from University of California found that an AI system trained on images labeled ‘pandas’ began seeing them in images where there were no pandas at all. The system saw giraffes and bicycles as pandas. Cute but concerning.
In fact, the term “AI hallucinations” is borrowed from psychology. The word “hallucination” is used for this phenomenon because of its similarity to what happens when humans experience hallucinations. Just like humans who, as a result of a mental or a neurological disorder, can see or hear things that aren’t there, AI tools generate responses that are not based on reality. It’s worth emphasizing that while human hallucinations are perceptions disconnected from the external world, AI hallucinations refer to confident responses that lack grounding in any of its training data.
How is the situation nowadays?
Let’s do a pulse check.
Where we are now
After experimenting with different tools, ChatGPT in particular, we must admit that the situation with AI hallucinations is getting better.
Remember the infamous example about France giving a TV tower to Lithuania? Here’s how ChatGPT answered me as of August, 2023:
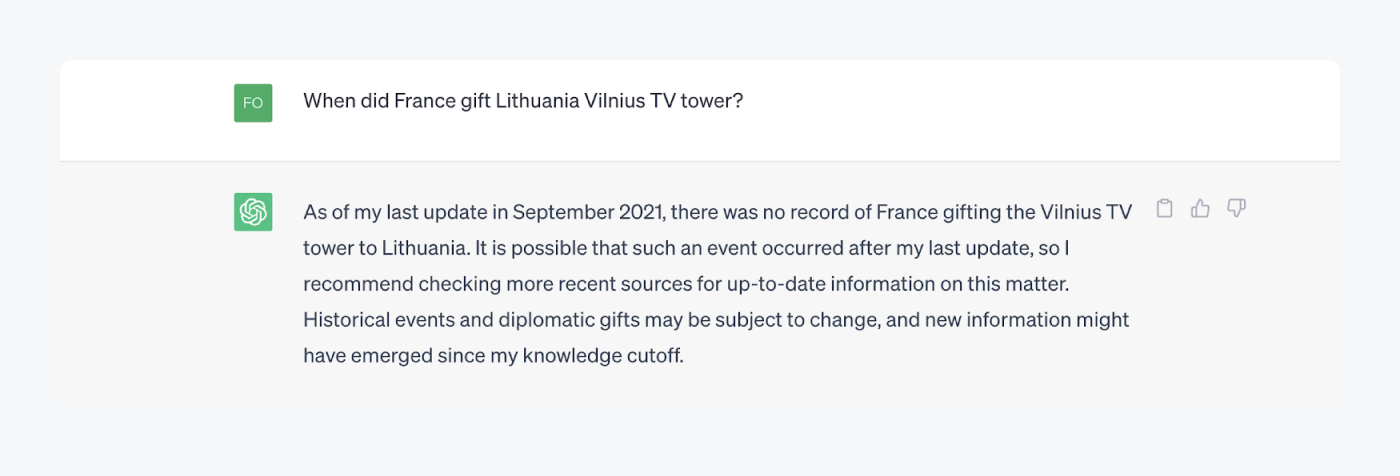
That’s good news. Companies are said to have taken actions to fight AI hallucinations in their LLMs. For example, OpenAI announced their new method for tackling the issue. The brand’s strategy is to train AI models to reward themselves for each correct step of reasoning when they’re moving towards an answer instead of just rewarding the end-answer.
This approach is called “process supervision” and is an alternative to “outcome supervision”. It has a potential to drastically lower the rates of hallucinations since the process will resemble more of a human chain of thought.
“The motivation behind this research is to address hallucinations in order to make models more capable at solving challenging reasoning problems."
![]()
In the research paper announcing this method, OpenAI has also released a dataset of 800,000 human labels that were used to train the new model.
Still, for now, it’s just research. LLMs learn from their interactions with users, and tech companies are scratching their heads to discover an antidote to these issues.
But what do the users think about all this?
Going back to our research, we must admit that companies do have to think hard about the solutions as the problem of AI hallucinations is widespread and worrisome.
Let’s take a closer look at some of the statistics discovered in our research.
About 96% of internet users know of AI hallucinations, and around 86% have personally experienced them
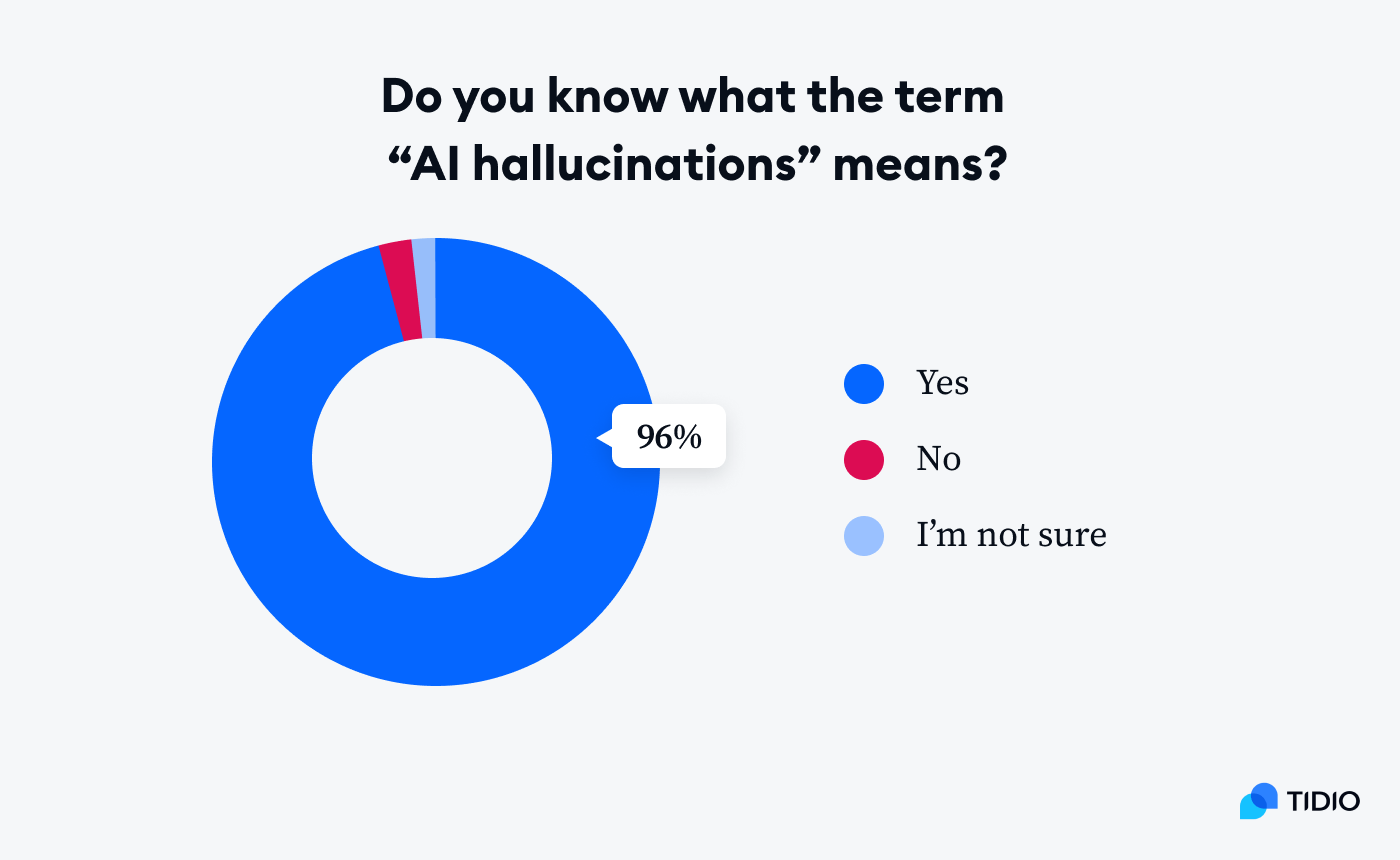
Even though the term has entered massive use only recently, it has spread like wildfire. People know about AI hallucinations, and most do so from personal experiences.
Users have encountered the issue in generative AI tools of all kinds: from ChatGPT and Bard through Siri and Alexa to Midjourney. How do they feel about them? Well—most people are intrigued, annoyed, or anxious. Not exactly positive news for AI developers.
Around 72% trust AI to provide reliable and truthful information, however, most of them (73%) have been misled by AI at least once
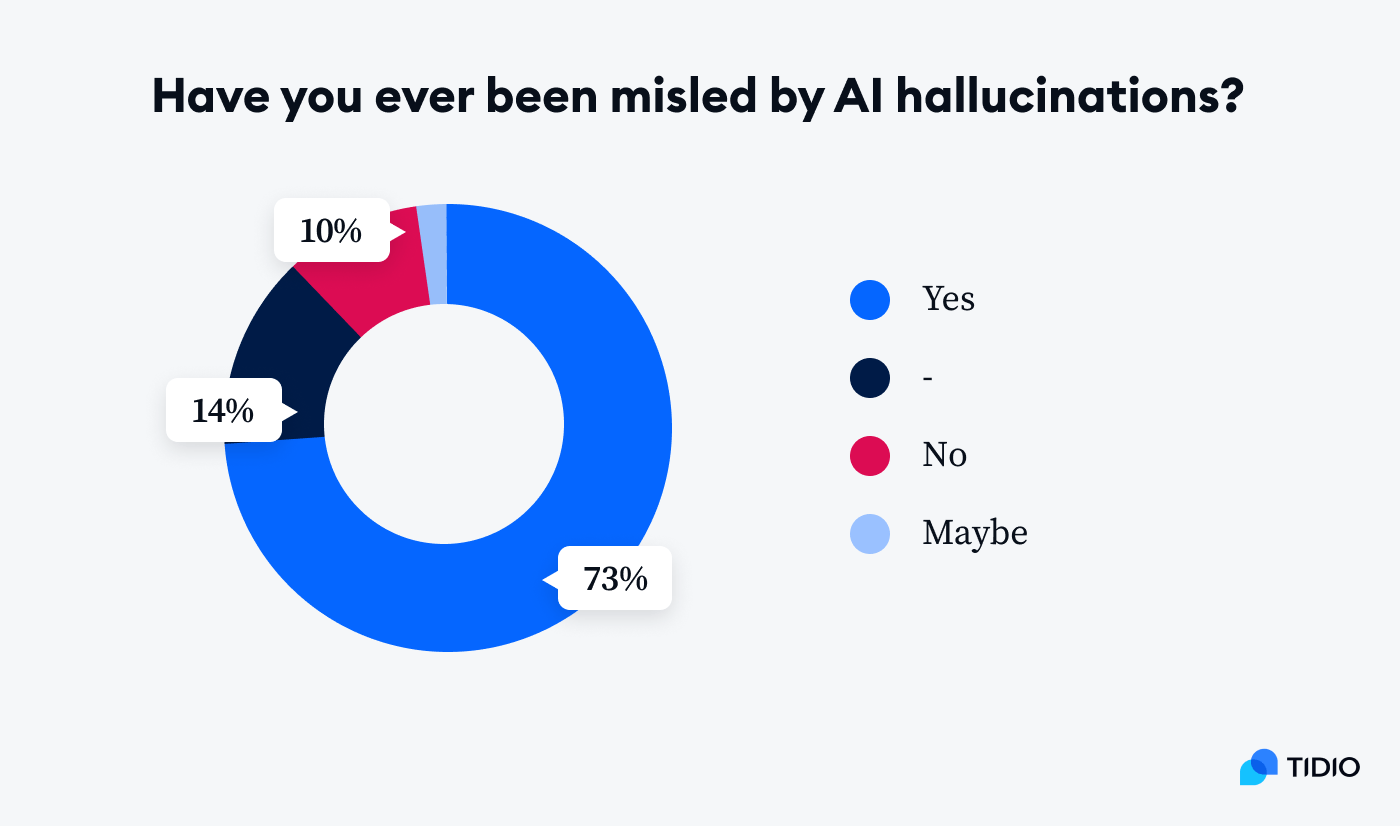
That stings. AI tools have a great reputation—people trust them and rely on them for their work and daily life. However, how can this trust not be undermined when the majority of users have been misled by AI hallucinations?
From harmless word mishaps to wrong math calculations to concerning factual inconsistencies—most of us have experienced those. With such numbers, it’s about time AI companies accelerate their efforts to minimize AI hallucinations as the trust levels in AI will only be dropping.
As many as 32% of people spot AI hallucinations by relying on their instincts, while 56% cross-reference with other resources
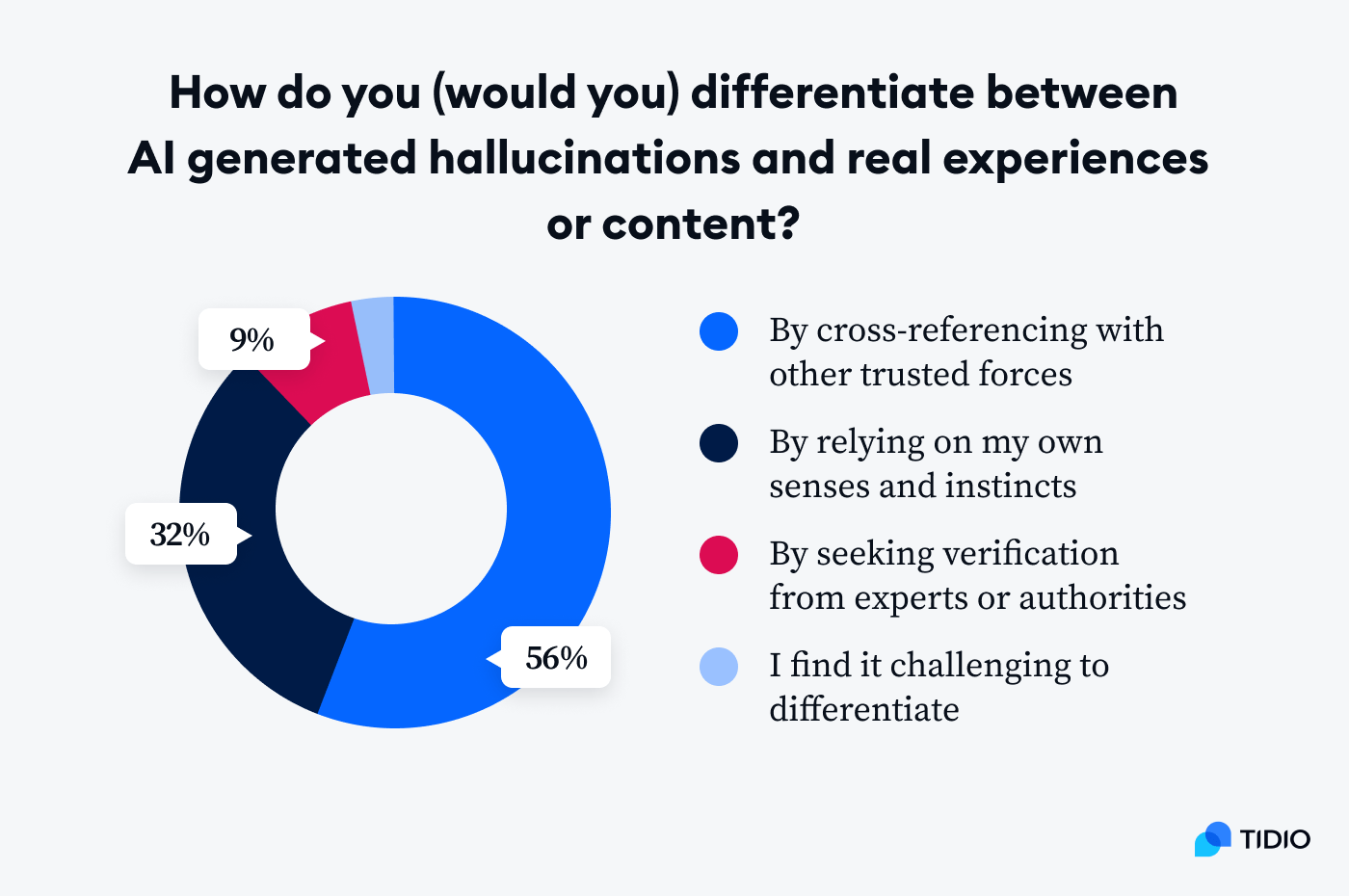
It’s great news that AI hallucinations can usually be spotted. When something doesn’t seem realistic (like the world record for crossing the English channel on foot), people either disregard such a response intuitively or cross-check with other resources.
However, unless actions are taken, AI hallucinations may become more intricate and harder to spot, which might mislead many more people. How do we manage this then?
About 47% would vote for stronger regulations and guidelines for developers
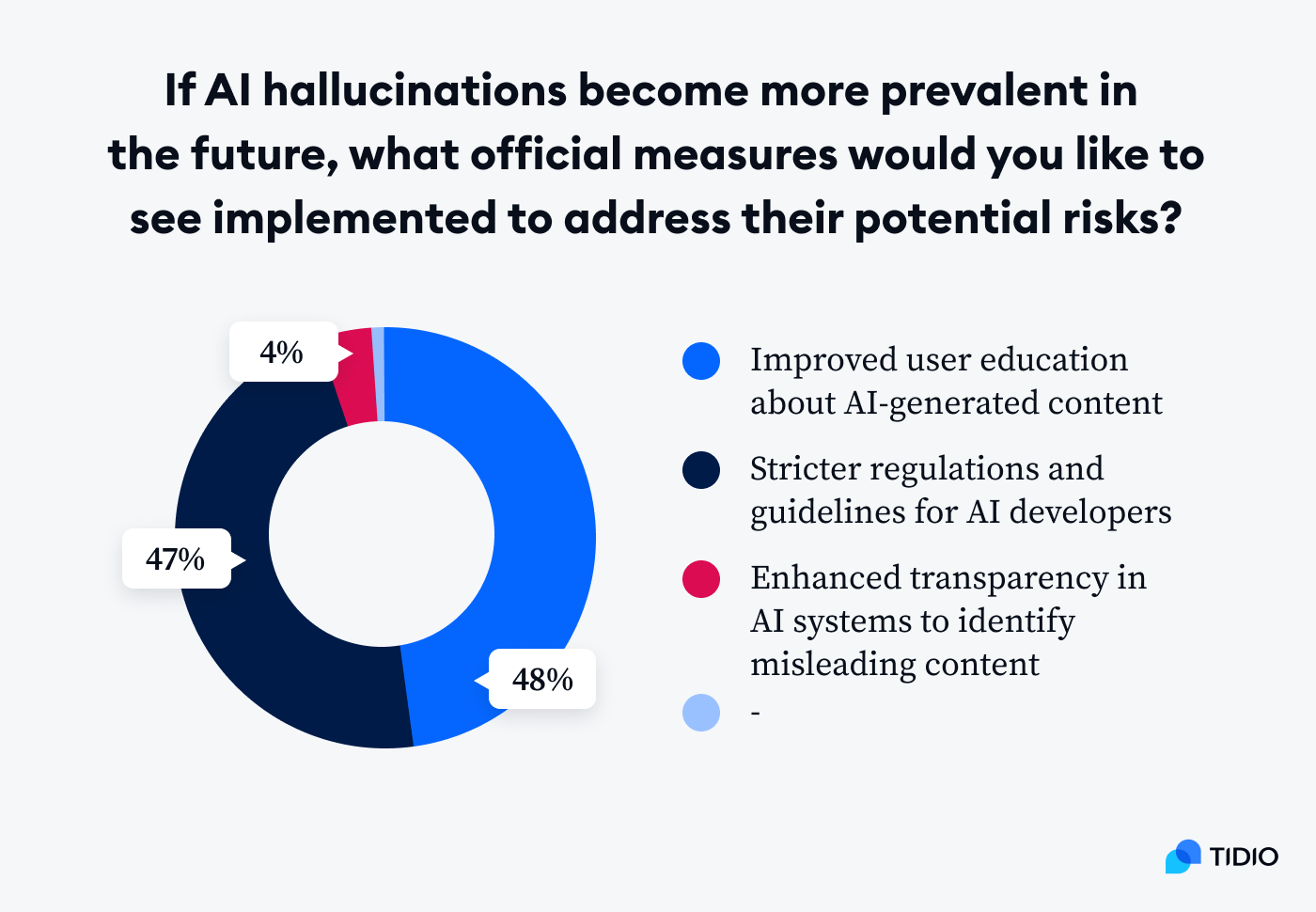
Almost half of the respondents want stricter regulations to be imposed on AI companies and businesses that take part in building LLMs and AI tools. It might make sense since mainstream tools like ChatGPT are used by all kinds of people, including kids (or criminals).
Having access to a powerful know-it-all tool is already a lot, but if, in addition to this, it’s prone to hallucinations, we’re in for some trouble. Stricter measures in place might help minimize potential issues.
Read more: Check out our research on the wide-scale generative AI adoption and what people think of it
Around 93% are convinced that AI hallucinations can harm the users
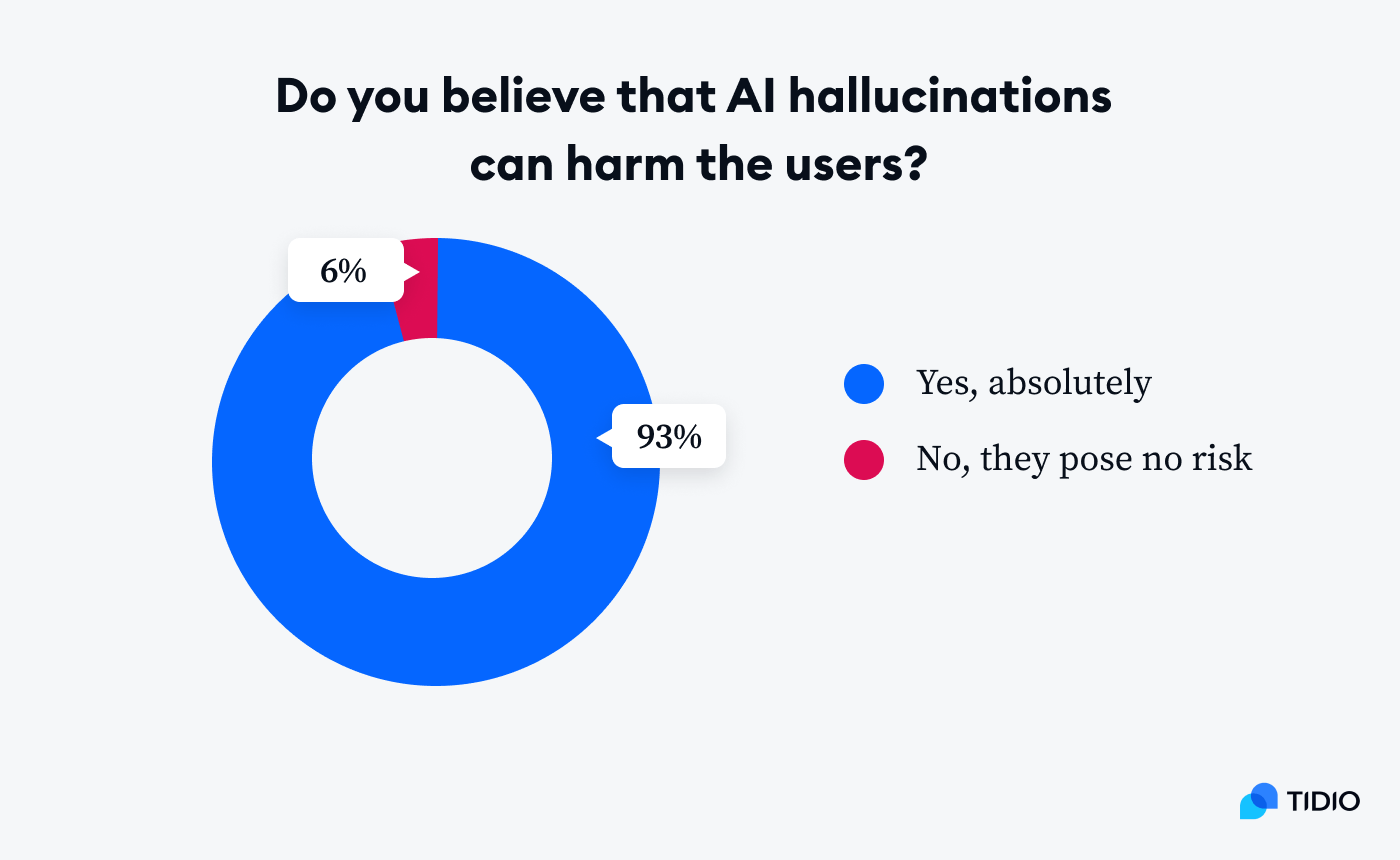
That’s a handful of concerned people. When 93% of users are sure that AI hallucinations have a potential to be harmful, it means it’s really serious.
However, ‘harm’ is a big word. What specific consequences can happen if AI hallucinations go out of control?
Read on to learn more about it.
Consequences of AI hallucinations
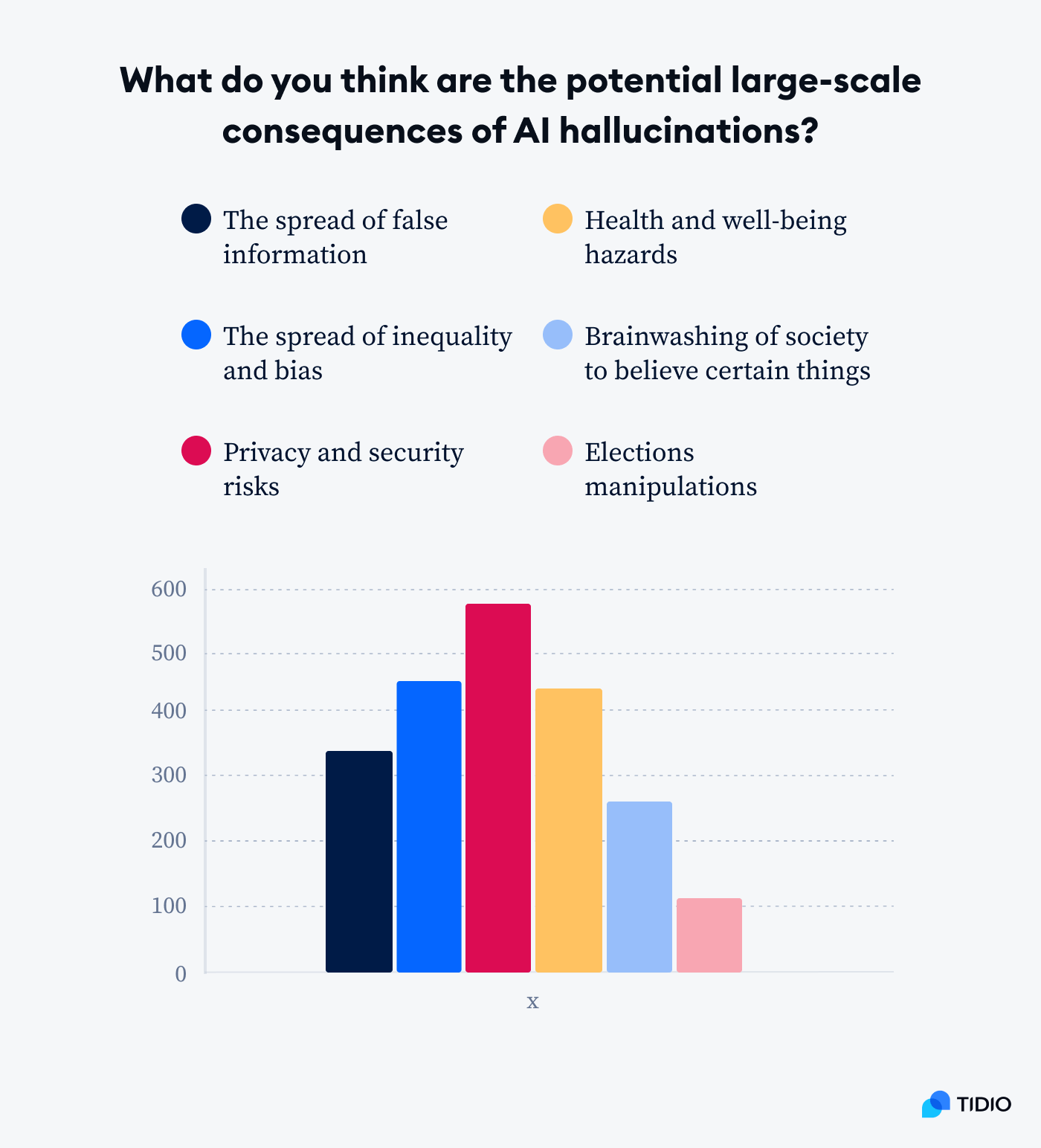
According to our respondents, the top three potential consequences of AI hallucinations include privacy and security risks (60%), the spread of inequality and bias (46%), and health and well-being hazards (44%). These are followed by the spread of false and misleading information (35%), brainwashing of society (28%), and even elections manipulations (16%).
Let’s get deeper into some of these fears and find out what can really happen if AI hallucinations go out of control.
Dissemination of false and misleading information
This is a valid fear. Fake information spreads like wildfire, and if it does on a massive scale, more and more people will be questioning their beliefs and perceptions. There are sectors where accuracy of data is of utmost importance like healthcare, finance, or legal services, so AI hallucinations can do a lot of harm there. This could result in health and well-being hazard risks that 44% of our respondents worry about.
The spread of bias and inequality
According to our research, generative AI is already pretty biased. As the example of Microsoft chatbot Tay that we discussed earlier shows, AI hallucinations can contribute to escalating the situation with biases and prejudice in AI tools. Still, AI hallucinations and AI biases are two different issues: biases in artificial intelligence are systematic errors in the system coming from data or algorithms that are biased. AI hallucinations are when the system cannot correctly interpret the data it has received.
Legal and compliance risks for companies
One third of businesses across all industries already use AI in some form, and the numbers are rising. If those AI tools hallucinate, businesses might be in for some trouble. The output of AI tools, if wrong, might cause real-world consequences, and businesses might have to deal with legal liability. Additionally, multiple industries have strict compliance requirements, so AI hallucinations make AI tools violate those compliance standards and lead to many losses for the business.
Right, AI hallucinations can be very negative for both the businesses and individuals. So, how can we minimize the potential harm in order to keep using the systems?
The first step is to learn to spot when AI hallucinates.
How to spot AI hallucinations
The good news is that for now, most AI hallucinations are spotted because they don’t pass the “common sense” test. AI will sound confident telling you about crossing the English channel on foot, however, it’s not hard to realize it’s impossible.
Still, there are some AI hallucinations that are harder to spot, especially when it comes to sources for something, works by real people, and simply not obvious facts. Sometimes, asking a follow-up question can help: the tool will apologize and say that it gave the wrong answer.
However, the best possible way to spot AI hallucination is doing your own fact-checking. If something is true, there is information about it in credible resources. So, it’s vital to remember not to take everything AI says at face value, but do your research and double-check. Our respondents seem to agree that cross-checking with credible resources is the best way to notice if something is off with AI responses.
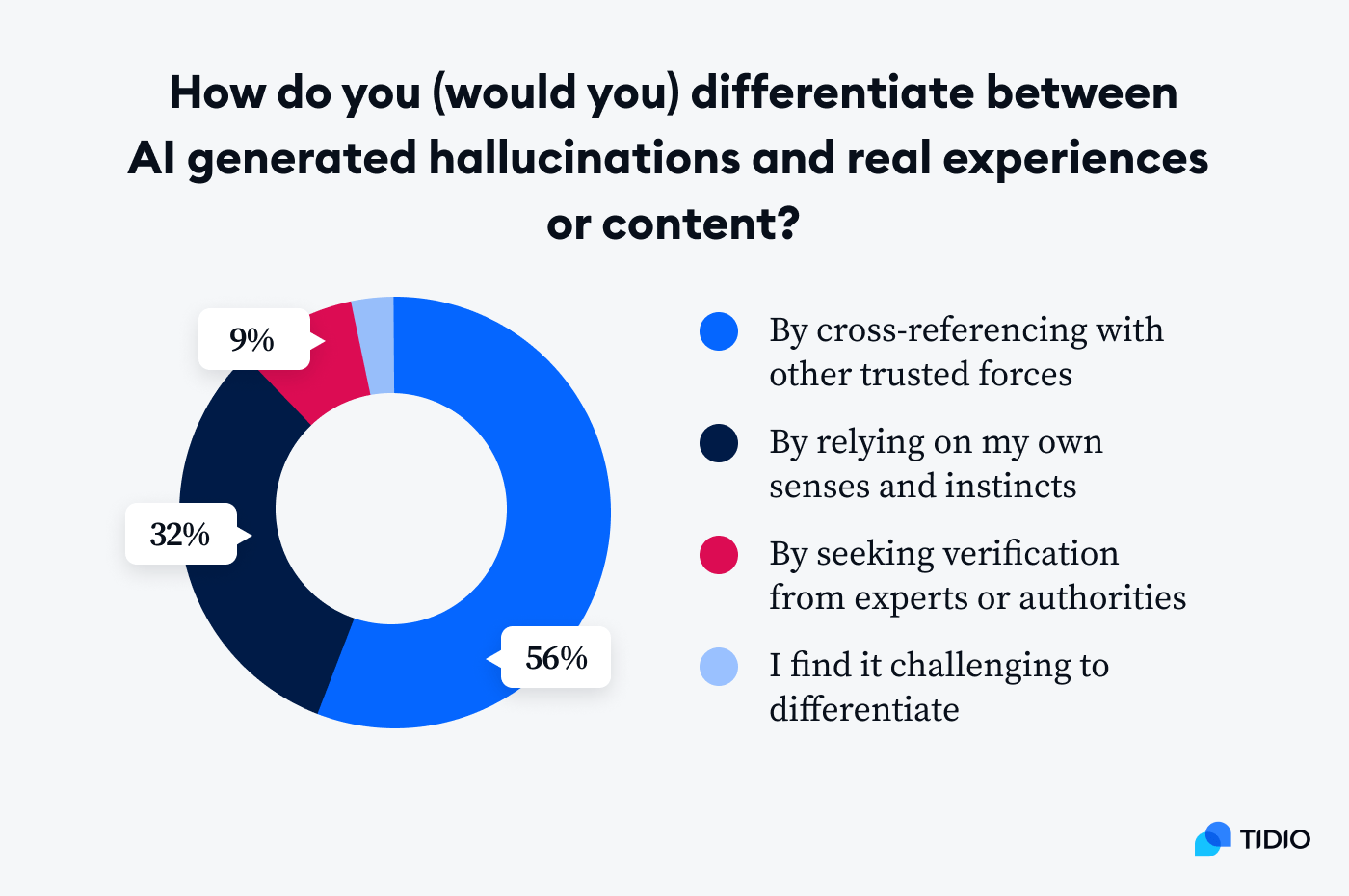
There are also ways to minimize the occurrence of hallucinations in your interaction with AI tools. Check out the tips in the next section.
How to prevent AI hallucinations
There are definitely ways which any regular user can use to minimize their risks of being misled by AI. Of course, they don’t guarantee that AI hallucinations will not happen, but from our experience, they definitely reduce their occurrence. Here are some tested best practices from us:
Be specific with your prompts
This is the surest way to get the output you want, without any unexpected results like AI hallucinations. Additional context in your prompt can be really helpful to limit possible outcomes and provide the tool with relevant data sources. An example of this is telling an LLM to imagine that it is something that can provide you with the information you need.
Another good tactic is to ask the tool to choose from a list of options instead of asking open-ended questions. In the end, multiple choice questions are easier at exams for a reason. Same with AI—a strict list of options prevents it from inventing things.
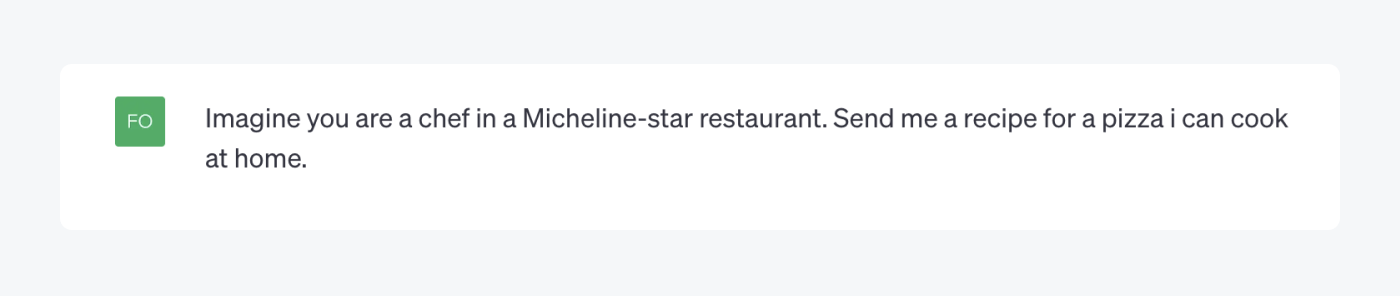
Filter the parameters
Most LLMs have a set of filters and parameters that you can play with. Something that can influence AI hallucinations is the Temperature parameter that controls the randomness of outputs. If the temperature is set higher, the outputs get more random.
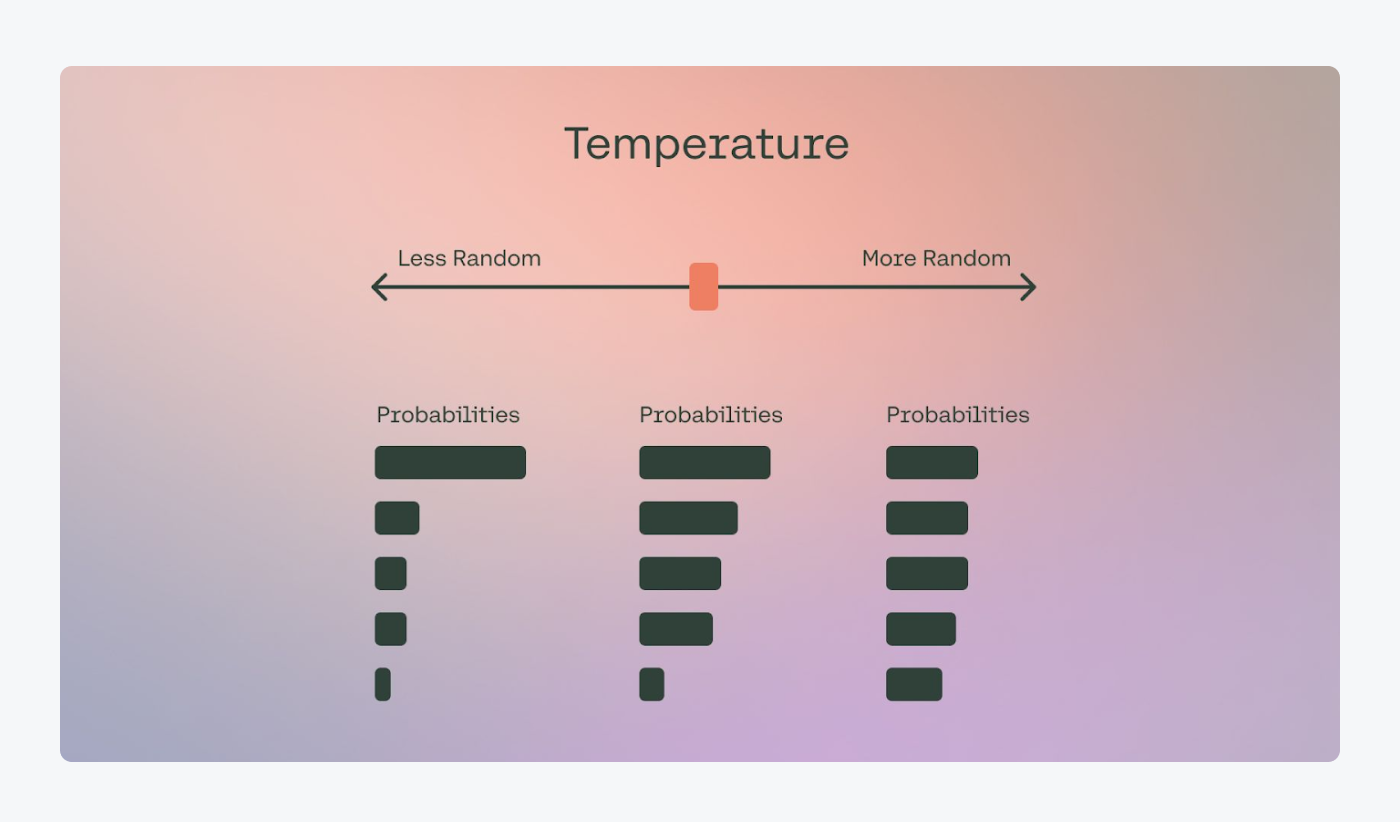
Use multishot prompting
LLMs don’t know words or sentences. They know patterns. They build sentences by assessing the probability of words coming after other words. So, to help them with this task, you can provide a few examples of your desired outputs. This way, the model can better recognize the patterns and offer a quality response.
Be cautious about using AI for calculations
As we already know, LLMs have their issues with math (just like humans do). Our advice is to be extremely careful when using AI for calculations.
LLMs are trained on large volumes of textual data, not mathematical data. So, they learn relationships between words, not numbers. Their core skills are around language processing, not arithmetic. While AI can learn to do simple arithmetic through training, it struggles with more complex mathematical reasoning. Its statistical foundations make AI better at pattern recognition in language, not manipulating numerical concepts. In short, AI can’t readily transfer arithmetic rules to new problems.
Advances in model architecture and training approaches may eventually help AI become better at calculations. However, currently, it remains a notable weakness compared to their language skills, and our tip is to avoid using AI for any kind of complex calculations.
Tell the tool what you don’t want
Letting AI know what output you don’t want to get is as important as what you want to get. That’s true! Preventing the tool from generating responses you don’t need is a great way to also prevent it from hallucinating (which, we assume, you also don’t need). Just ask AI to exclude certain facts or data, and you are good to go.
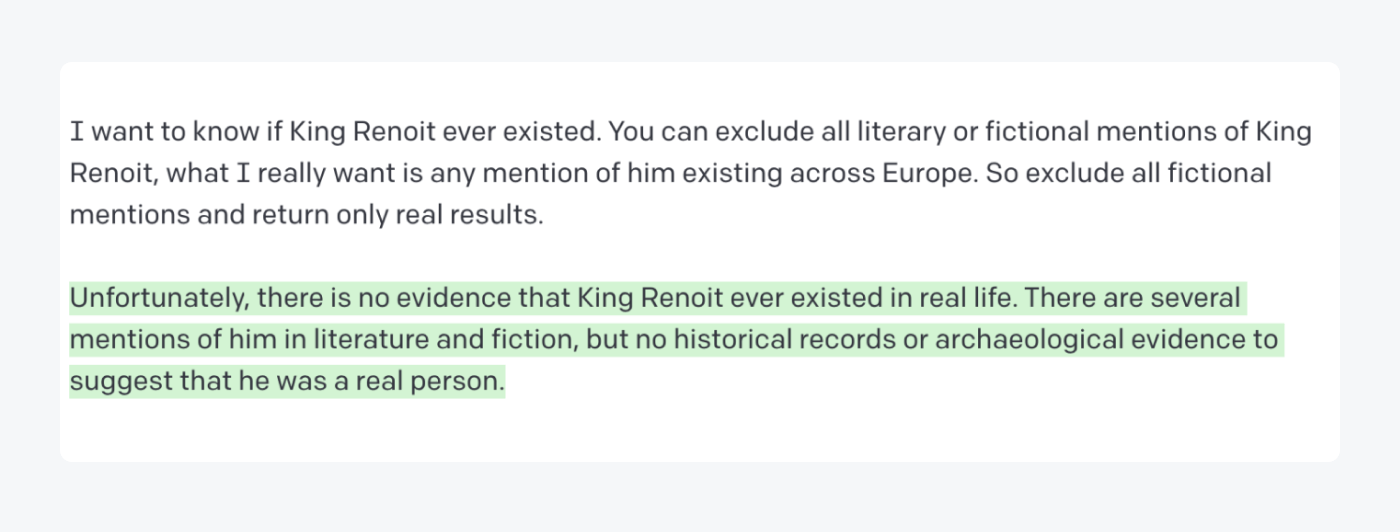
Give feedback to AI
Just like humans, AI learns from experience. In the case of generative AI tools, they learn from their interactions with users. When AI gives a false response or starts to hallucinate, it doesn’t know what’s happening, and that’s where you can help.
Respond that the tool’s answer is not correct or helpful. If you are using ChatGPT, you can press the feedback button to notify the tool that the response wasn’t helpful. This way, it will learn from your communication, and hopefully, improve.
As you can see, there are definitely ways to prevent AI hallucinations. Of course, we should always be aware of them and stay alerted that something we read might not necessarily be true. Just like anywhere on the internet. But that’s another story.
For now, let’s summarize the takeaways from this research.
AI hallucinations: key takeaways
Nothing in the world is perfect, including AI.
It makes mistakes, confuses things, and suffers from AI hallucinations. People who use AI tools know about the issue, and they are definitely worried. Hopefully, this can be a sign to companies that action needs to be taken. Otherwise, the consequences might be dire.
The good news is that there are definitely ways to spot and prevent AI hallucinations. Even better news is that the tools are getting better. It seems like we’re moving in the right direction.
However, it’s vital to be vigilant and strive not to fall victim to AI hallucinations. In the end, humans will always be smarter than robots, so we can’t let them fool us. Fact-checking and seeking the truth are our biggest weapons. And now is the time to use them.
Sources
- Generative AI hallucinations: Why they occur and how to prevent them
- How to Tell When an Artificial Intelligence Is ‘Hallucinating’
- AI Hallucinations: Understanding the Phenomenon and Exploring Potential Solutions
- Hallucinations of ChatGPT-4: Even the most powerful tool has a weakness
- The hilarious & horrifying hallucinations of AI
- What are AI hallucinations and how do you prevent them?
- AI hallucination
- Chatbots sometimes make things up. Is AI’s hallucination problem fixable?
- Survey of Hallucination in Natural Language Generation
- Hallucinations in Neural Machine Translation
- History of generative AI innovations spans 9 decades
- OpenAI is pursuing a new way to fight A.I. ‘hallucinations’
- When A.I. Chatbots Hallucinate
- Titanic
- What Is AI Hallucination, and How Do You Spot It?
- ChatGTP and the Generative AI Hallucinations
- ChatGPT: What Are Hallucinations And Why Are They A Problem For AI Systems
- Both humans and AI hallucinate — but not in the same way
- Proceedings Fourth IEEE International Conference on Automatic Face and Gesture Recognition
- AI Biases: Are We Ready to Be StereotChat GPT-3 Statistics: Is the Future Already Here? [2023]yped by Robots?
- ChatGPT ‘may make up facts,’ OpenAI’s chief technology officer says
- Chat GPT-3 Statistics: Is the Future Already Here? [2023]
- 10+ Essential AI Statistics You Need to Know for 2023
Methodology
For this study about AI hallucinations, we collected responses from 974 people. We used Reddit and Amazon Mechanical Turk.
Our respondents were 71% male, 28% female, and ~1% non-binary. Most of the participants (55%) are Millennials aged between 25 and 41. Other aged groups represented are Gen X (42%), Gen Z (1%), and Baby boomers (6%).
Respondents had to answer 17 questions, most of which were multiple choice or scale-based ones. The survey had an attention check question.
Fair use statement
Has our research helped you learn more about AI hallucinations? Feel free to share the data from this study. Just remember to mention the source and include a link to this page. Thank you!